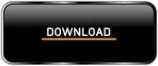

Xiawei Guo, Yuhan Quan, Huan Zhao, Quanming Yao, Yong Li, Weiwei TuĭCN V2: Improved Deep & Cross Network and Practical Lessons for Web-scale Learning to Rank Systems TabGNN: Multiplex Graph Neural Network for Tabular Data Prediction GNN on top decision trees from the GBDT algorithmīaseline: CatBoost, LightGBM, GAT, GCN, AGNN, APPNP, FCNN, FCNN-GNN TabGNN Net-DNF: Effective Deep Modeling of Tabular Dataīoost then Convolve: Gradient Boosting Meets Graph Neural Networks Ĭriteo AI Lab, Yandex HSE University MIPT
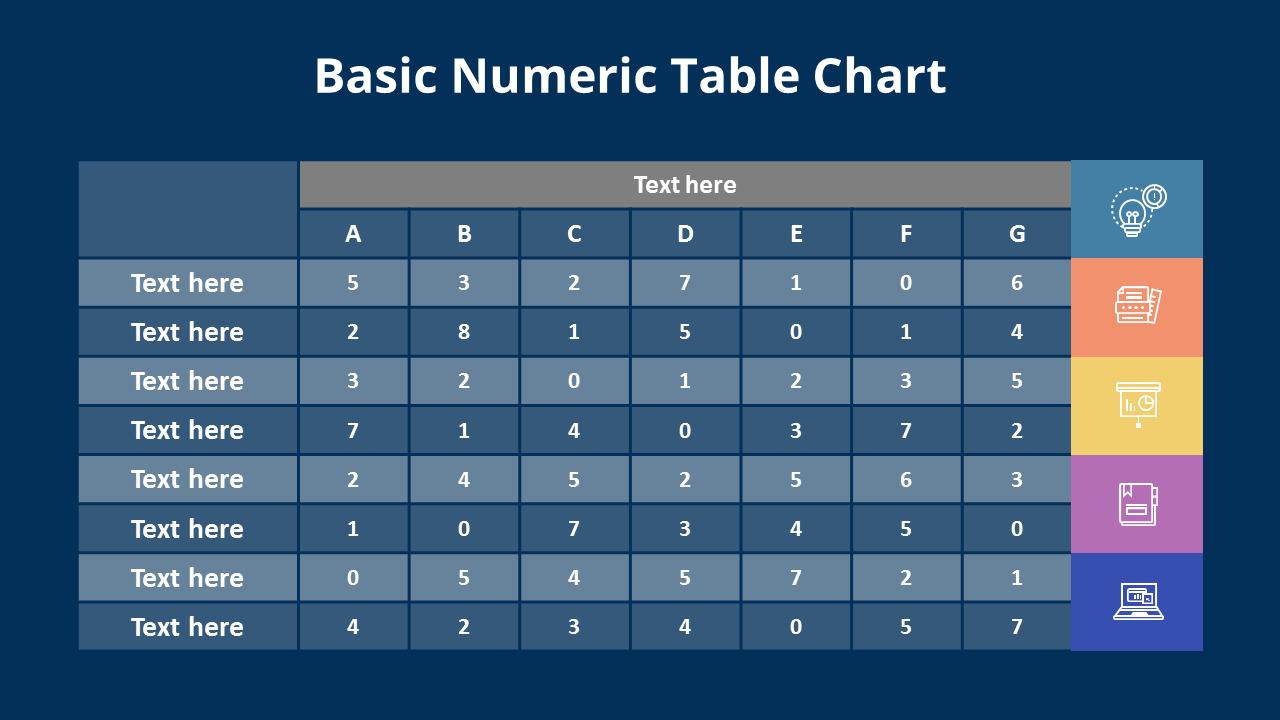
Use Bi-GRU and GCN to simultaneously extract spatial and relational information from tables.īaseline: SVM, CART, FCNN-MT, TAPAS Net-DNF MSRA, Beijing Normal University, Peking University Lun Du, Fei Gao, Xu Chen, Ran Jia, Junshan Wang, Jiang Zhang, Shi Han, Dongmei Zhang TabularNet: A Neural Network Architecture for Understanding Semantic Structures of Tabular Data Jiarui Qin, Weinan Zhang, Rong Su, Zhirong Liu, Weiwen Liu, Ruiming Tang, Xiuqiang He, Yong Yuįully exploits both cross-row and cross-column patterns.īaseline: FM models, GBDT, IPNN, PIN, FGCNN TabularNet Retrieval & Interaction Machine for Tabular Data Prediction Reconstructing the data from the subset of its features rather than its corrupted version in an autoencoder setting can better capture its underlying latent representation.īaseline: XGBoost, AE, DAE, CAE, VIME-self RIM Respiratory and Immunology, R&D, AstraZeneca Talip Ucar, Ehsan Hajiramezanali, Lindsay Edwards SubTab: Subsetting Features of Tabular Data for Self-Supervised Representation Learning NUS, Zhejiang University, University of Michigan, Beijing Institute of TechnologyĪdaptive relational modeling with multi-headgated attention networkīaseline: FM models, AFM, HOFM, DCN, CIN, AFN, DNN, GCN, GAT, AFN+ SubTab Shaofeng Cai, Kaiping Zheng, Gang Chen, H. Ioannidis, Soji Adeshina, Yangkun Wang, Tom Goldstein, David Wipfīaseline: GAT, GCN, AGNN, APPNP, CatBoost, Boost-GNN ARM-NetĪRM-Net: Adaptive relational modeling with multi-headgated attention network Sajad Darabi, Shayan Fazeli, Ali Pazoki, Sriram Sankararaman, Majid Sarrafzadehīaseline: CatBoost, Manifold Mixup, VIME EBBSĬonvergent Boosted Smoothing for Modeling Graph Data with Tabular Node Features SCARF: Self-Supervised Contrastive Learning using Random Feature Corruptionĭara Bahri, Heinrich Jiang, Yi Tay, Donald Metzlerīaseline: Methods of label noise or data augmentation Contrastive MixupĬontrastive Mixup: Self- and Semi-Supervised learning for Tabular Domain Bayan Bruss, Tom Goldsteinīaseline: XGBoost, LightGBM, CatBoost, MLP w.

Gowthami Somepalli, Micah Goldblum, Avi Schwarzschild, C.
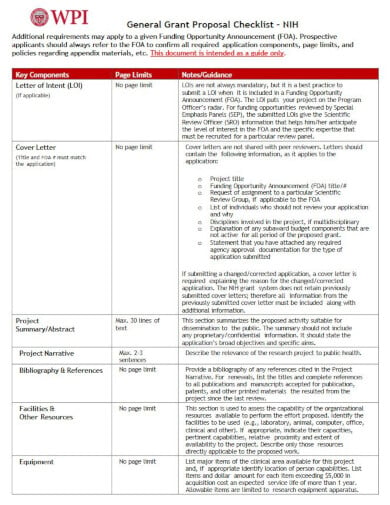
SAINT: Improved Neural Networks for Tabular Data via Row Attention and Contrastive Pre-Training Chen, Jian WuĮxplicitly group correlative input features and generate higher-level features for semantics abstraction.īaseline: XGBoost, CatBoost, gcForest, Net-DNF, TabNet, NODE, FCNN SAINT Jintai Chen, Kuanlun Liao, Yao Wan, Danny Z. Data Mining DANetsĭANETs: Deep Abstract Networks for Tabular Data Classification and Regression Jiaxuan You, Xiaobai Ma, Daisy Yi Ding, Mykel Kochenderfer, Jure Leskovecįeature imputation is formulated as an edge-level prediction task and the label prediction as a node-level prediction task in a GNN. Handling Missing Data with Graph Representation Learning QCRI, HBKU, Qatar, Renmin University GRAPE Nan Tang, Ju Fan, Fangyi Li, Jianhong Tu, Xiaoyong Du, Guoliang Li, Sam Madden, Mourad Ouzzani RPT: Relational Pre-trained Transformer Is Almost All You Need towards Democratizing Data Preparation Hiroshi Iida, Dung Thai, Varun Manjunatha, Mohit IyyerĪ self-supervised pretraining approach trained exclusively on tables by asking the model to predict whether or not each cell in a table is real or corrupted. TABBIE: Pretrained Representations of Tabular Data Learn task-agnostic contextualized representations Yury Gorishniy, Ivan Rubachev, Valentin Khrulkov, Artem Babenkoĭata mining: extracting information from a specific row with observed attributes, i.e., predicting labels with features.ĭata preparation: addressing with missing or dirty value of rows, i.e., estimating features with or without label.Ĭolumn population, row population, and column type prediction Revisiting Deep Learning Models for Tabular Data The University of Tübingen, Tübingen, Germany Vadim Borisov, Tobias Leemann, Kathrin Seßler, Johannes Haug, Martin Pawelczyk, Gjergji Kasneci Deep Neural Networks and Tabular Data: A Survey
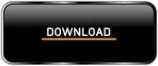